Harnessing the Power of Deep Learning Chips in AI Development
Written on
Chapter 1: Introduction to Deep Learning Chips
As artificial intelligence (AI) evolves, the hardware supporting it must keep pace. This brings us to deep learning chips, which are engineered to perform the intricate mathematical calculations required for deep learning—an essential method in AI. These specialized chips not only enhance the power of AI but also increase its speed and efficiency, making them crucial for the future of AI advancements.
So, what are deep learning chips and why are they vital? Let’s delve deeper.
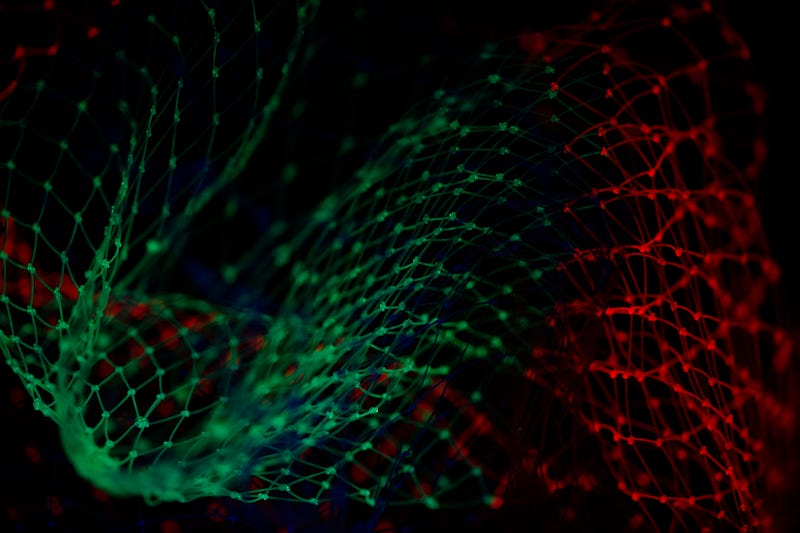
Chapter 1.1: Understanding Deep Learning Chips
Deep learning chips are microprocessors specifically crafted for deep learning tasks. They are integral in constructing and training neural networks—algorithms inspired by the human brain that learn and identify patterns.
The significance of deep learning chips lies in their ability to manage the vast amounts of data essential for training these networks. Furthermore, they operate at speeds far exceeding those of conventional microprocessors, making them ideally suited for deep learning tasks.
Chapter 1.2: The Impact of Deep Learning Chips on AI
Your smartphone exhibits intelligence, capable of voice recognition and command responses. However, it operates on shallow learning, excelling at specific tasks like face recognition or text translation but lacking the ability to adapt those skills to new contexts.
In contrast, deep learning allows machines to learn various tasks autonomously. This approach mimics human brain functionality, and it’s the advent of deep learning chips that is propelling AI's capabilities to unprecedented levels. These chips excel at processing large datasets rapidly, enabling quick learning of new tasks and the ability to apply them in diverse situations. Thus, AI is becoming smarter and faster, thanks to the innovation of deep learning chips.
Chapter 2: The Fundamentals of Deep Learning Chips
Deep learning chips serve as a form of artificial intelligence that creates and interprets patterns. Their applications range from facial recognition to natural language processing.
There are two primary varieties of deep learning chips: CNN (convolutional neural network) chips, which are optimal for image recognition, and RNN (recurrent neural network) chips, designed for tasks like speech recognition and natural language processing.
Chapter 2.1: Advantages of Deep Learning Chips
The advantages of utilizing deep learning chips are vast. These chips can swiftly and accurately process extensive datasets, which is critical for advanced machine learning tasks. This capability opens the door to developing intricate AI systems, such as autonomous vehicles that can recognize objects and respond in real time.
Additionally, deep learning chips facilitate AI applications that function autonomously, such as voice assistants and chatbots, which necessitate significant computational power.
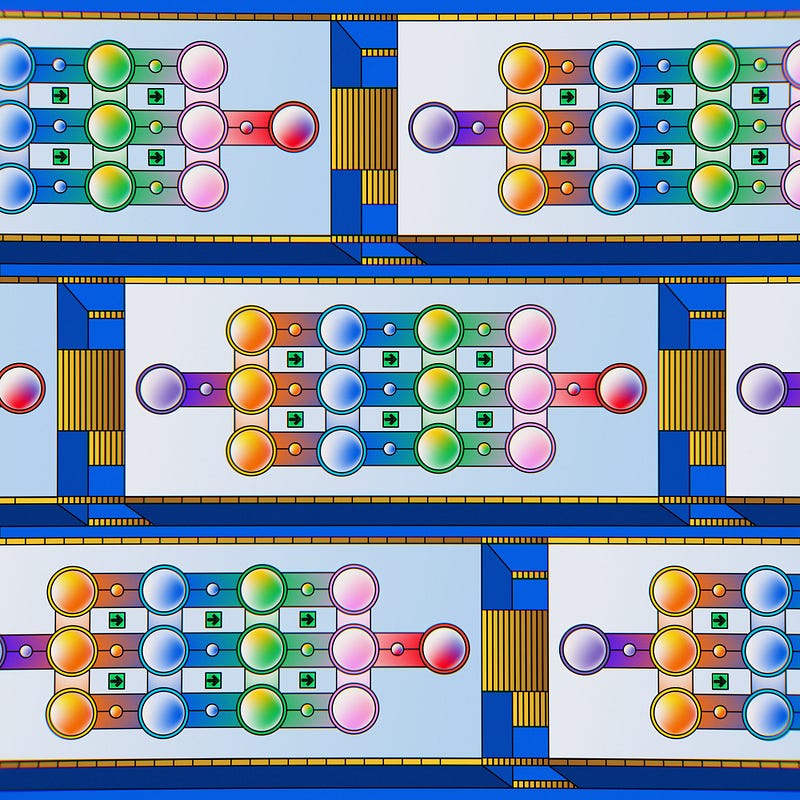
Moreover, these chips consume less power, making them ideal for IoT devices where battery longevity is a priority. Their compact size also allows for integration into embedded systems where space is a constraint.
In summary, deep learning chips hold tremendous promise for developing powerful AI technologies that could transform our world.
Chapter 3: Common Deep Learning Chips in Use
Currently, a variety of deep learning chips are employed in AI applications, including six predominant types: GPUs, TPUs, FPGAs, ASICs, EGUs, and Neuro Processors.
Beginning with GPUs—these are the most commonly used chips, excelling in parallel computing for complex matrix operations and handling high-dimensional data inputs. Next are TPUs, or tensor processing units, which are Google’s proprietary chips optimized for machine learning algorithms.
FPGAs (field-programmable gate arrays) allow users to adjust hardware configurations on the chip for performance optimization. In contrast, ASICs (application-specific integrated circuits) are tailored for specific functions like facial recognition.
EGUs (enhanced graphics unit processors) provide improved performance and image quality over GPUs and FPGAs. Lastly, Neuro Processors can accommodate more sophisticated neural networks than other prevalent deep learning chips.
Chapter 4: Challenges and Considerations
Despite their advantages, utilizing deep learning chips presents challenges. A major hurdle is accessing the appropriate data; chips require quality data for effective learning, necessitating time and resource investment in data collection and validation.
Additionally, it’s vital to consider whether the end product can be tailored for individual users. If customization isn’t feasible, scalability issues may arise.
Finally, ensuring that your system facilitates transparent and explainable decision-making is crucial. While deep learning models are highly accurate, their complexity often renders them opaque, complicating the understanding of their decisions. Developing an AI system that can audit its own decisions, rather than relying solely on human oversight, is advisable.
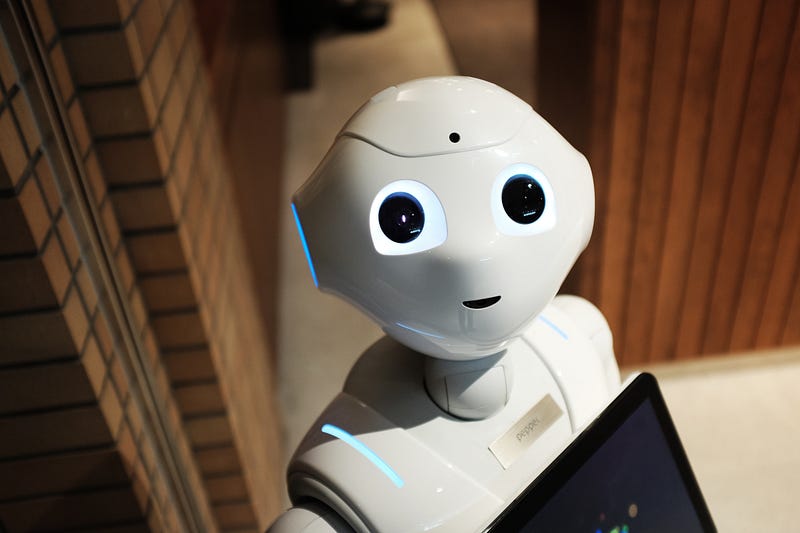
Chapter 5: Conclusion
What does this mean for you and your organization? To stay competitive and effectively incorporate AI into your business strategy, investing in deep learning chips is essential.
Chip manufacturers are increasingly recognizing the importance of deep learning, with plans to release numerous chips specifically designed for this purpose. This trend is promising for businesses, as it suggests that the costs associated with deep learning will continue to decrease.
Now is the ideal time to consider investing in deep learning chips if you wish to integrate AI into your business operations.
This video titled "Using Large Chips for AI Work" explains how advanced chips are revolutionizing AI performance and efficiency.
The second video titled "How Chips That Power AI Work | WSJ Tech Behind" delves into the mechanics behind AI chips and their significance in technology.