Effective Healthcare Data Visualization Using Python Libraries
Written on
Chapter 1: Introduction to Data Visualization
In this article, we explore the fundamentals of visualizing healthcare data using two prominent Python libraries: Matplotlib and Seaborn. Both libraries are highly regarded in the data science and analytics community for their capabilities.
Matplotlib: This library excels at creating basic plots with a high degree of customization. It integrates seamlessly with pandas and numpy, making it straightforward to create multiple figures.
Seaborn: Known for its aesthetic appeal, Seaborn is a robust tool for data visualization. It works well with pandas DataFrames and offers beautiful themes for your plots. However, it may occasionally lead to Out Of Memory (OOM) issues when handling large datasets.
To illustrate the capabilities of Matplotlib and Seaborn, we will examine several visualization techniques below.
Section 1.1: Visualizing Data with Matplotlib
To start visualizing data, we must first import the necessary libraries:
import pandas as pd
import matplotlib.pyplot as plt
Next, we load the healthcare data:
# Reading the healthcare dataset
df = pd.read_csv('healthcare.csv')
To view the first few rows of the data, we can use:
df.head()
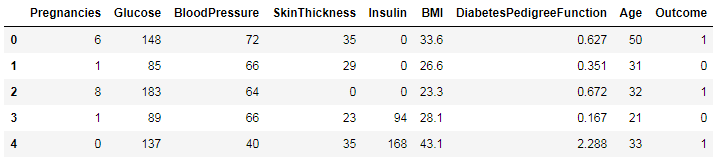
Boxplot Analysis
Boxplots are essential for visualizing the quartiles and identifying outliers within the data.
for column in df:
if df[column].dtype in ['int64', 'float64']:
plt.figure()
df.boxplot(column=[column])

Histogram Visualization
Histograms are utilized to analyze the distribution of the dataset:
df.hist()
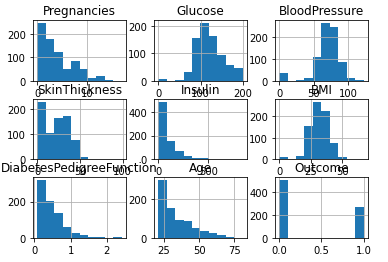
Scatter Plot Creation
Scatter plots allow us to examine the relationship between two variables. For instance:
x = df['Age']
y = df['Glucose']
plt.scatter(x, y)
plt.xlabel('Age')
plt.ylabel('Glucose')
plt.title('Age vs Glucose')
plt.show()
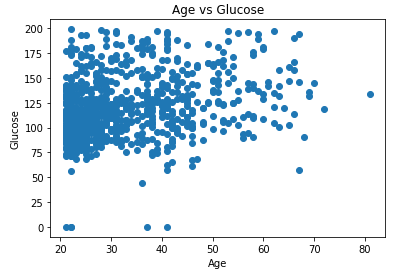
Bar Plot for Categorical Data
Bar plots are effective for visualizing the counts of categorical variables:
plt.bar(x, y)
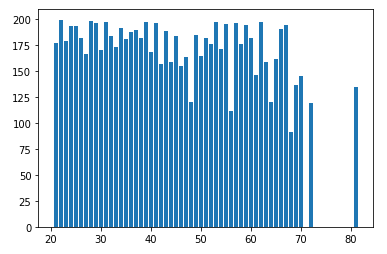
Chapter 2: Advanced Visualization Techniques
The first video titled "Data Visualization with Matplotlib and Seaborn (Python)" provides insights into effectively utilizing these libraries for data visualization.
In the second video, "How to Visualize Data in Python Using Seaborn," you will learn advanced techniques for using Seaborn to enhance your data visualizations.
Seaborn Library Overview
After importing Seaborn, we can create more sophisticated plots. For example, a joint plot combines a histogram and scatter plot:
import seaborn as sns
sns.jointplot(x=x, y=y, data=df, size=5)
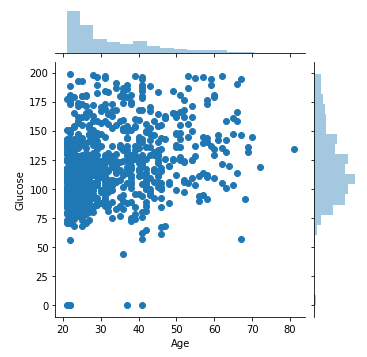
Boxplot and Stripplot in Seaborn
We can also create boxplots and overlay them with strip plots for a more detailed view:
sns.boxplot(x="Outcome", y="Age", data=df)
sns.stripplot(x="Outcome", y="Age", data=df, jitter=True, edgecolor="gray")
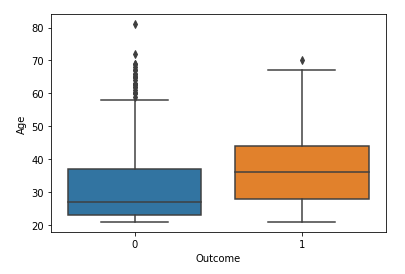
Conclusion
Visualizing data is a powerful technique for understanding relationships between features and deriving insights. While many plotting options are available, this article highlighted a selection that can significantly enhance your analysis.
I hope you found this article helpful. Feel free to connect with me on LinkedIn or Twitter for further discussions.
Recommended Articles
- NLP — Zero to Hero with Python
- Understanding Python Data Structures
- Exception Handling in Python
- Principal Component Analysis Explained
- An In-depth Guide to K-means Clustering
- Comprehensive Overview of Linear Regression
- Understanding Logistic Regression
- Basics of Time Series Analysis
- Data Wrangling Techniques in Python
- Exploring the Confusion Matrix in Machine Learning