# AI Mastering Ecology Through StarCraft II Gameplay
Written on
Chapter 1: The Rise of AI in Gaming
The journey of artificial intelligence in gaming began with Deep Blue's victory over chess champion Garry Kasparov in the late '90s. Since that moment, AI and machine learning systems have continually tackled new challenges in the gaming world. However, some games present more significant hurdles than others. The ancient game of Go, for example, posed a considerable challenge due to its reliance on intuition and complex strategies. A 2001 paper noted that the challenges of Computer Go necessitated innovative AI problem-solving techniques, making it a compelling research area.
Fast forward to 2015, when AI AlphaGo triumphed over the legendary Go player Lee Sedol, securing a 4-1 victory while displaying unconventional and creative strategies.
Section 1.1: Enter StarCraft II
StarCraft, a science fiction real-time strategy game, has established itself as one of the most popular and intricate games in the genre. Until recently, it was regarded as the next monumental challenge for artificial intelligence. Enter AlphaStar, a sophisticated deep neural network trained using raw gameplay data through supervised and reinforcement learning techniques. In a remarkably short time, AlphaStar outperformed a top human team in StarCraft II. Before long, it achieved ‘Grandmaster’ status, a rank reserved for the elite 0.02% of players who have mastered the game.
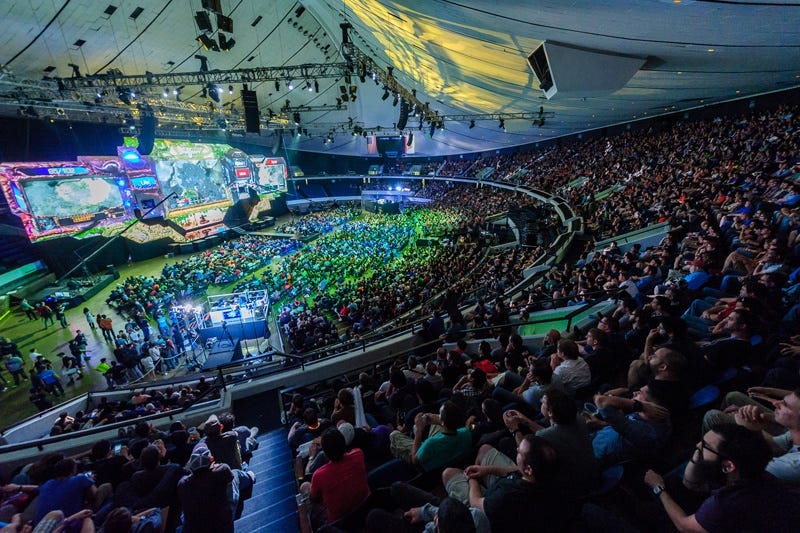
Section 1.2: Gameplay Dynamics and Ecology
In StarCraft, players control three distinct ‘races’ competing for resources and territory:
- Protoss (akin to high-tech space elves): Slow to build but extremely powerful.
- Zerg (resembling insect-like creatures): Rapidly reproduce, though most units are weak.
- Terrans (humans with advanced technology): A balanced approach between the two.
This environment, characterized by resource scarcity and diverse strategies, mirrors the dynamics of an ecosystem. A recent study explored these ecological principles within the game. Players typically start with an ‘r-strategy,’ focusing on quickly producing numerous low-cost units to establish dominance. However, as the game advances, strategies shift toward a ‘K-strategy,’ where players invest in fewer but more specialized and costly units.
AlphaStar adeptly navigated this game-ecology overlap. Trained on data from top human players, it also developed its own unique strategies. Some of these unconventional methods involved overloading resource collection or maintaining a uniform army composition. Remarkably, these tactics often led to swift victories, highlighting AlphaStar’s profound grasp of ecological principles.
The first video, "Machine Learning AI (DI-Star) Can Beat StarCraft II Pros," showcases the remarkable capabilities of AI in mastering complex gaming environments, including its strategic innovations.
Chapter 2: Ecological Insights from AI Strategies
The authors of the study propose utilizing StarCraft and AlphaStar to further investigate real-world ecological and evolutionary patterns. By manipulating virtual environments, resource distribution, and the types of species involved, we could uncover insights into their evolutionary paths. This approach may shed light on how various species adapt to changing conditions, such as climate change and invasive species.
For plants, these findings could inform us about their responses to environmental shifts. For animals, particularly humans, it may enhance our understanding of interpersonal dynamics and cultural interactions.
The second video, "A Guide to DeepMind's StarCraft AI Environment," delves into the AI's training environment and its implications for understanding complex systems, including ecological interactions.
In an era where I used to study ecology through textbooks, the intersection of AI and ecology in games like StarCraft II offers a fresh, dynamic perspective on understanding natural systems.